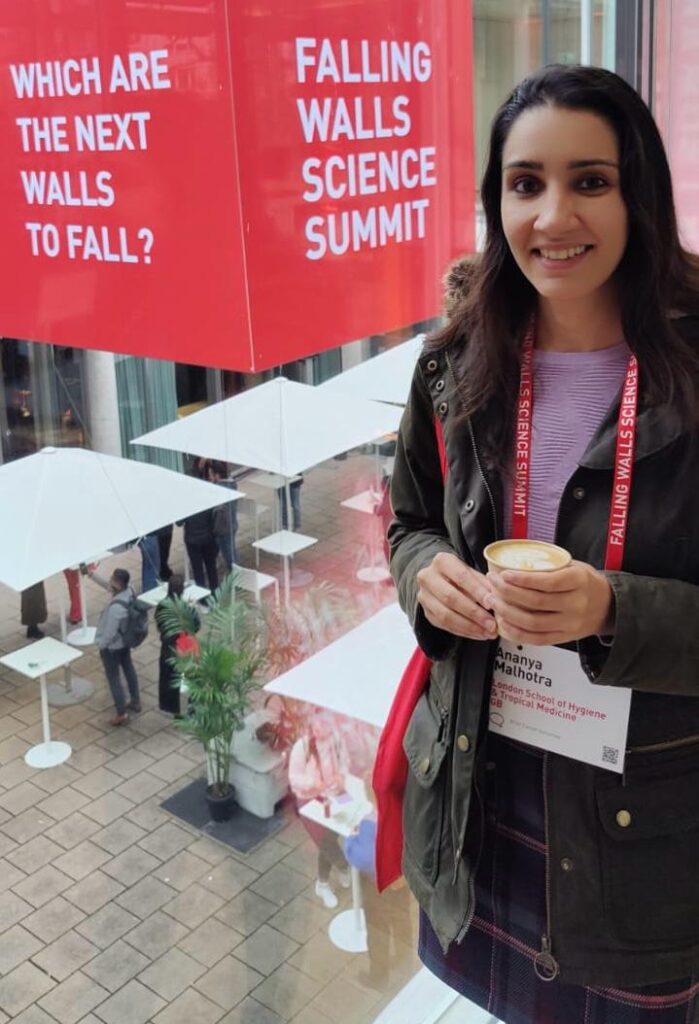
This year, as the world marked the 35th anniversary of the fall of the Berlin Wall, I had the privilege of attending the Falling Walls Science Summit 2024 in Berlin. The conference brought together global science leaders, business pioneers, and public sector visionaries to exchange ideas and explore innovative solutions to some of the world’s most pressing challenges, all with a focus on creating a positive impact on humanity.
Among the many compelling presentations, one in particular resonated deeply with me: a talk on the cutting-edge applications of artificial intelligence (AI) in cancer treatment. The session focused on Onconaut, an AI-powered tool developed at the Max Delbrück Center in Berlin. This innovative system leverages AI to guide oncologists and patients toward personalised cancer care by rapidly analysing complex medical data. Onconaut addresses a critical challenge in oncology: the sheer volume of diagnostic tests and treatment options, which can often overwhelm clinicians and delay timely decision-making.
The presentation struck a personal chord as it closely parallels my ongoing research on early detection of pancreatic cancer using machine learning tools. My work involves analysing electronic health records in primary care settings across England, searching for subtle symptom patterns that could lead to earlier and potentially life-saving diagnoses. Like Onconaut, my approach harnesses AI to decode complex datasets, aiming to improve outcomes for patients who might otherwise be diagnosed too late for curative treatment.
In addition to my research on pancreatic cancer, Onconaut’s goals align with those of another project I am involved in: QUALITOP. This European initiative is developing an immunotherapy-specific open Smart Digital Platform to aggregate real-world data from clinical centres in four EU countries. By integrating lifestyle, genetic, and psychosocial factors, the platform monitors health status and quality of life (QoL) for cancer patients undergoing immunotherapy. Using big data analysis, machine learning, and simulation modelling, QUALITOP aims to deliver real-time, personalised recommendations tailored to individual patient profiles.
What stood out to me during the talk was how Onconaut exemplifies AI’s transformative potential in oncology. By analysing cancer biomarkers, clinical guidelines, and patient data, the tool generates tailored recommendations in seconds, bridging the gap between groundbreaking research and clinical practice. This capability is particularly vital for cancers like pancreatic cancer, where late diagnosis often limits treatment options. An AI-powered approach to early detection could be life-saving, offering patients the chance for curative treatments such as surgery.
AI as a Partner in Medicine
Beyond its practical applications, the talk highlighted a broader trend: the rise of AI as a partner in medicine, complementing rather than replacing human expertise. Tools like Onconaut are not designed to replace oncologists but to empower them by making complex decisions more efficient and accessible. This vision aligns with my own work in primary care, where AI can augment clinicians’ abilities to detect cancers earlier and ensure equitable access to these advancements for all populations.
The Bigger Picture: AI’s Role in Oncology
The integration of AI into oncology heralds an exciting new era. From diagnosing and treating cancers to monitoring patient outcomes, tools like Onconaut and QUALITOP’s platform demonstrate how big data and machine learning can address the complexity of modern cancer care. However, these innovations also bring challenges. Ethical concerns such as data privacy, algorithmic bias, and the need for rigorous validation must be addressed. Furthermore, ensuring equitable access to AI-driven solutions is crucial to avoid exacerbating existing health disparities.